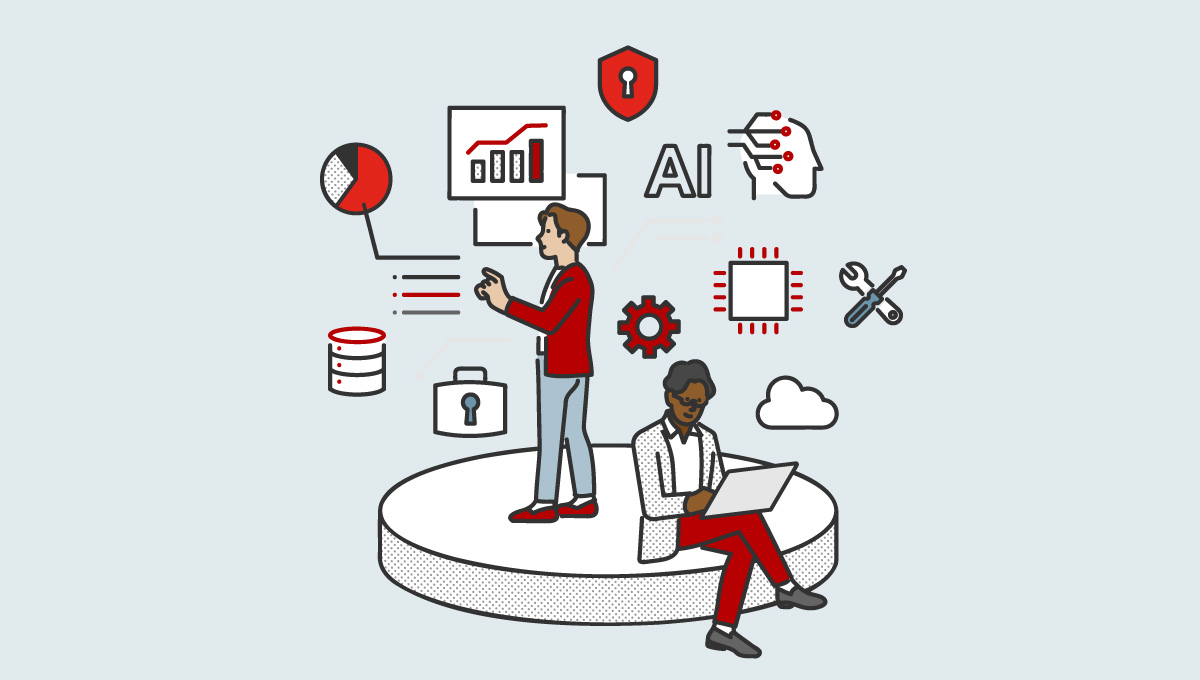
Artificial Intelligence (AI) often seems like an overnight success story, but its roots stretch back more than 80 years.
The journey from the inception of neural networks to the sophistication of ChatGPT is a fascinating one, marked by significant milestones, technological advancements, and cultural shifts. Let’s explore how we got here and why it didn’t happen sooner.
AI’s 80-Year Journey
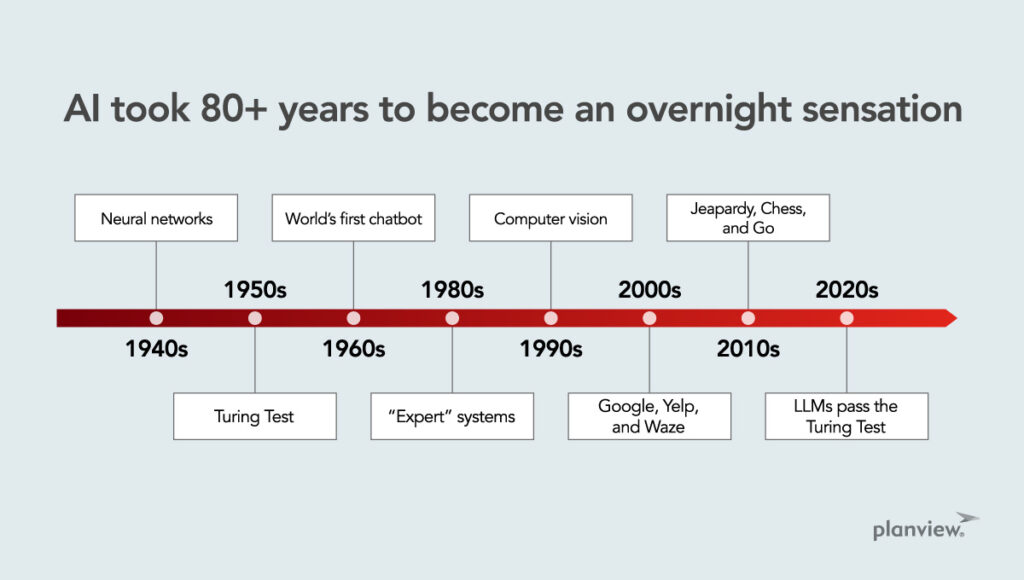
The early days: Neural networks and the Turing Test
In 1948, the concept of neural networks was introduced, laying the groundwork for AI. By 1950, Alan Turing’s seminal paper “Computing Machinery and Intelligence” posed the famous Turing Test, asking if a machine could be indistinguishable from a human in conversation. This question would guide AI development for decades.
The first chatbot: Eliza
The year 1966 saw the unveiling of Eliza, the world’s first chatbot, at MIT. Designed as a “computer therapist,” Eliza was simplistic, often parroting users’ statements. Intended as a demonstration to show the banality and limits of current technology in the sixties, Eliza captivated many, hinting at the seductive potential of conversational AI.
The rise and stumble: “Expert” systems and early AI
The 1980s witnessed attempts at creating expert systems for use in legal, healthcare, and financial industries. Even the boldest of these experiments were narrow in their expertise and prohibitively expensive to train, falling far short of the hype and tainting the AI label.
However, the 1990s saw neural networks finding practical applications in computer vision and character recognition, as well as other areas where modest-sized neural networks were applicable, reigniting interest in AI.
The era of machine learning
The 2000s marked the beginning of machine learning infiltrating our lives through applications like Google, Yelp, and Waze. This period set the stage for AI’s integration into daily life, making the technology more accessible and familiar to the general public.
Dominating games: AI’s triumphs in Jeopardy, Chess, and Go
The 2010s were highlighted by AI algorithms conquering human champions in Jeopardy, Chess, and Go. These victories demonstrated AI’s growing capabilities and its potential to surpass human expertise in specific domains. But they also served as a warning, as the breakthroughs that developed these capabilities were slow to transfer to domains like healthcare, despite billions spent on research.
The history of AI is not just about technological advancements but also about cultural shifts, economic factors, and human curiosity and adaptability.
The advent of large language models
The 2020s saw large language models like ChatGPT passing the Turing Test, a milestone that redefined AI’s conversational abilities. This era also proposed a new challenge: That an AI should be capable of creating a startup worth $1M in two months, displaying AI’s potential in entrepreneurship.
The Four Cs Behind AI’s Evolution
Like many technological advances, there is no single cause of AI’s ascent. I propose that the following four drivers, applied over the last 30 years, can explain how 2023 became the year AI became ubiquitous.
Compute Capabilities and Cost
Moore’s Law played a crucial role, enhancing computing capabilities and making parallel computing feasible, albeit still with significant costs involved in training and operating large language models (LLMs).
Culture
The ubiquity of smartphones accustomed us to algorithms in our daily lives, from navigation to recommendations, paving the way for AI’s acceptance.
Corpus
The internet provided an unprecedented repository of digitized text, crucial for training LLMs, albeit not without controversy regarding data usage.
Conversation
While less than one-half of one percent of all people on earth know how to code, we all begin acquiring language skills at around six months of age. Our innate language skills made language-based AI particularly appealing, leading to rapid adoption and viral growth.
The Final Catalysts: Courage and Cash
Starting in 2016, a combination of courage and financial backing led researchers to scale neural networks to unprecedented levels. The launch of GPT-4 in March 2023 epitomized this trend, showing that larger models could reach beyond their remarkable linguistic abilities and display evidence of careful reasoning.
A Journey of Incremental Advances and Bold Leaps
The history of AI is not just about technological advancements but also about cultural shifts, economic factors, and human curiosity and adaptability. From the early days of neural networks and the creation of the (daunting) Turing Test to the era of ChatGPT, AI’s journey is a tapestry of incremental advances, bold leaps, and well-intentioned missteps.
Time will tell as to whether large language models will herald a new age for AI.
As we look to the future, it’s important to remember that, in some ways, we’ve been here before – at least we thought so at the time. Here are some examples.
- The term “robots” and a warning of their dangers first appeared in “R.U.R.”, a play by Czech author Karel Čapek in 1920.
- In 1965, Nobel Laureate Economist Herb Simon – often cited as one of a handful of theoreticians who paved the way for AI – warned that within 20 years, machines would be capable of any task performed by humans.
- Five years later, Marvin Minsky (another AI trailblazer) claimed that we were less than a decade away from a machine equal to humans in intelligence.
- Five- and ten-year forecasts for AI throughout the aughts and teens were almost unanimous in their optimism about trillion-dollar productivity improvements, but those predictions proved overblown.
Time will tell as to whether LLMs will herald a new age for AI, where each of us has constant access to a hyper-efficient, empathetic, and tireless personal assistant, physician, and life coach. Though we seem closer than ever, there are real challenges around compute cost, compute speed, hallucinations, and accuracy.
The only certainty is the ongoing race to build the AI we’ve been promised for over a century will not be boring.
Read more on AI from Dr. Sonnenblick:
- The Revolution is Here: Generative AI and Project Management
- Our AI Strategy (and a Blueprint for Yours)
- Trends in AI: Should You View Data as a Product?
Catch what Planview’s CTO, Dr. Mik Kersten, says about Planview’s Copilot capabilities and productivity with AI: